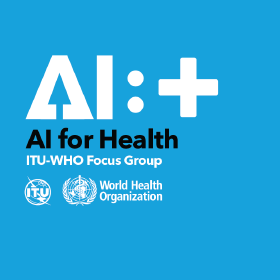
On 19 October, 2023, the World Health Organization, in collaboration with the International Telecommunication Union, released a draft listing key regulatory considerations for AI in healthcare (“Considerations”). This draft provides six considerations for regulating AI used in healthcare, and recommendations for enacting such regulations. The WHO communicated in its intent behind these Considerations that innovation must be retained while effective regulations are put in place for governing AI systems.[1]
We will take a look into these Considerations, and analyse them in comparison with other AI regulations that cater to healthcare.
BACKGROUND
The WHO and ITU began a joint effort to regulate AI by establishing a focus group called the Focus Group on “Artificial Intelligence for Healthcare” (FG-AI4H), in 2018. The FG-AI4H focuses on publishing guidelines for the use of AI in various healthcare fields. In June, 2022, the FG-AI4H published Ethics and Governance for AI in Health (“Ethical Principles”),[2] which lays down ethical principles for the use of AI in healthcare. This guidance also focuses on how existing laws pertaining to human rights and data protection can be interpreted to bring AI within their scope. The ethical principles laid down under this guidance include the protection of human autonomy, promoting human well-being and safety, and public interest, ensuring transparency, explainability, and intelligibility, ensuring responsibility and accountability, inclusiveness, and equity, and promoting responsible AI.
Regulatory Considerations on AI for Health
While the Considerations also serve as a guidance document, similar to the Ethical Principles, the Considerations go a step further to provide actionable steps toward AI governance. These include considerations for lawmakers while drafting AI policies or regulations, or adapting existing laws to regulate AI.
Documentation and Transparency. In order to maintain transparency in the AI system, the draft suggests that regulations include a provision for documentation that should be maintained during the life cycle of the AI system. This includes documentation from the point of ideation and conception to the point of development of the AI system and after its deployment. A risk-based approach that identifies errors, biases, and the level of documentation required must be considered.
Risk Management and AI Systems Development Lifecycle Approach. The draft suggests a risk-based approach that must be considered throughout the lifecycle of an AI system, especially during the pre and post-mark development stages. It emphasizes the adoption of a holistic risk evaluation and management, that takes into account the complete context in which the AI system is intended to be used. During the development stage, the requirement for developers to prioritize safety must be considered. Post-market development risks that include serious public health threats, death or serious deterioration in the health of a patient, or any device modification, exchange, or destruction, must be reported.
Intended Use and Analytical and Clinical Validation. Defining the intended use is of extreme importance, as the use cases of AI systems in healthcare vary depending on the context, ensuring that the end-user utilizes the AI tool safely. Thus, regulations that require clinical and analytical validation to assess the sufficiency of the AI system with the intended use, are suggested. Regulators may also consider overseeing the dataset used for training the AI systems. In countries such as the UK, where the NHS has a vast amount of clinical data, regulators may consider giving access to train AI systems on their databases. Such requirements for clinical and analytical validation could be carried out after the deployment and marketing of the AI system, and for the entirety of its lifecycle thereafter.
Data Quality. The draft suggests the classification of the quality of data into ten categories, which include, Volume (size of the data), Veracity (accuracy of the data), Validity (Data quality, governance, master data management), Vocabulary (semantics that describe the structure of data), Velocity (speed at which data is generated), Vagueness (Confusion over meaning of big data and tools), Variability (dynamic behaviour of data), Venue (data obtain from several platforms), Variety (different types of data), and Value (usefulness of the data). These attributes must be considered to assess the quality of the dataset for training the AI system and to ensure that bias, inaccuracy in output, and discrimination do not reflect in the AI tools.
Privacy and Data Protection. Consideration for enacting effective data protection regulations, ensuring documentation and transparency regarding the cybersecurity and privacy risks, and requiring AI regulatory sandboxes to ensure that the AI systems comply with data protection regulations, are emphasized in this draft.
Engagement and Collaboration. Regulators may consider creating platforms that provide accessible development and information, among the key stakeholders in AI innovation, manufacture, and deployment. The regulators may also consider engaging and collaborating with AI stakeholders, in order to ensure streamlining the process of oversight and accelerate the advances in AI.
Since the Ethical Principles were published in June, 2022, just before the AI wave hit with ChatGPT, it failed to identify several issues in AI systems. While it identifies issues regarding transparency, human well-being, and explainability, issues such as bias, discrimination, quality of data, and risk management are not addressed. Now that the stakeholders have a better insight into the risks involved in AI systems, the Considerations have addressed them with emphasis on all the issues.
ANALYSIS OF THE CONSIDERATIONS
Given that it is challenging to gauge the functionality of the AI systems, and how they arrive at the outputs, the WHO has placed emphasis on ensuring that the entire process of creating an AI system, right from its conception to its usage, is transparent and documented. This takes higher importance for AI systems used in healthcare, which will presumably be used by patients and healthcare providers. The Considerations also include the identification of attributes such as race, gender, religion, and ethnicity, that are intentionally representative, are reported.
These Considerations differ from existing regulations, such as the EU AI Act,[3] which is currently pending its final draft. While it categorises AI systems using a risk-based approach, it also creates a category of unacceptable risk, high-risk, and foundational models. The high-risk systems that include AI systems meant for health insurance, contain several obligations and requirements, including risk management, data governance, human oversight, monitoring and record-keeping obligations, and standards for accuracy, robustness, and cybersecurity. Fines imposed under the EU AI Act for failure to comply with obligations range from EUR 10 million to 40 million, or 2%-7% of the annual turnover, depending on the severity of the violation. SMEs seem to be the most affected entities by such penalties, making the EU AI Act the most restrictive piece of AI legislation so far. Although the Considerations suggest similar obligations for developers, the document does not include provisions for penalizing violators.
The Indian Council of Medical Research (ICMR) released Ethical Guidelines for Application of Artificial Intelligence in Biomedical Research and Healthcare,[4] which aims to provide non-binding guidelines for the use of AI in biomedical research and clinical trials. The Guidelines require defining the intended use of the AI system, description of the broad principles of the AI system and its applications, notification for sources of funding and investment, ensuring the validity of the credentials of the developers of the AI system, process of recruitment of participants, risk management strategies, validation strategies, accountability, monitoring, collection and storage of data, informed consent, and data protection. These Guidelines work on similar lines to the Ethical Principles, both of which aim to allow innovation while ensuring responsible AI.
The Considerations are part of the few guidelines introduced to govern AI in the field of healthcare. Although the guidelines produced by the FG-AI4H do not introduce significant concepts for AI governance, they harmonize basic principles introduced globally, which include transparency and algorithmic bias, risk management, maintaining the quality of input data, collaboration among stakeholders, and data privacy. Most of these ideas are covered in the draft of the EU AI Act, which is the first piece of legislation that aims to regulate AI.
CONCLUSION
Given the ambiguity in the complications stored in AI neural networks, having general regulations in place that offer balanced global standards makes it easier for innovators to collaborate and communicate, and create AI systems that are safe for use. The Considerations act as a roadmap to developing AI regulations that serve this purpose.
Authors: Shruti Gupta, Shantanu Mukherjee
References:
[1] https://iris.who.int/handle/10665/373421
[2] https://www.who.int/publications/i/item/9789240029200
[3]https://eur-lex.europa.eu/legal-content/EN/TXT/?uri=celex%3A52021PC0206
[4]https://main.icmr.nic.in/sites/default/files/upload_documents/Ethical_Guidelines_AI_Healthcare_2023.pdf